Prediction of Draft Force of a Chisel Cultivator Using Artificial Neural Networks and Its Comparison with Regression Model.
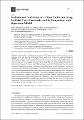
View/ Open
Date
2020-02Author
Abbaspour_Gilandeh, Yousef
Fazeli, Masoud
Roshanianfard, Ali
Hernández Hernández, Mario
Gallardo Bernal, Iván
Hernández-Hernández, José Luis
Metadata
Show full item recordAbstract
In this study, artificial neural networks (ANNs) were used to predict the draft force of a rigid tine chisel cultivator. The factorial experiment based on the randomized complete block design (RCBD) was used to obtain the required data and to determine the factors affecting the draft force. The draft force of the chisel cultivator was measured using a three-point hitch dynamometer and data were collected using a DT800 datalogger. A recurrent back-propagation multilayer network was selected to predict the draft force of the cultivator. The gradient descent algorithm with momentum, Levenberg¿Marquardt algorithm, and scaled conjugate gradient descent algorithm were used for network training. The tangent sigmoid transfer function was the activation functions in the layers. The draft force was predicted based on the tillage depth, soil moisture content, soil cone index, and forward speed. The results showed that the developed ANNs with two hidden layers (24 and 26 neurons in the first and second layers, respectively) with the use of the scaled conjugate gradient descent algorithm outperformed the networks developed with other algorithms. The average simulation accuracy and the correlation coecient for the prediction of draft force of a chisel cultivator were 99.83% and 0.9445, respectively. The linear regression model had a much lower accuracy and correlation coecient for predicting the draft force compared to the ANNs.
Collections
- Artículos Open Access [395]